residualization & projection
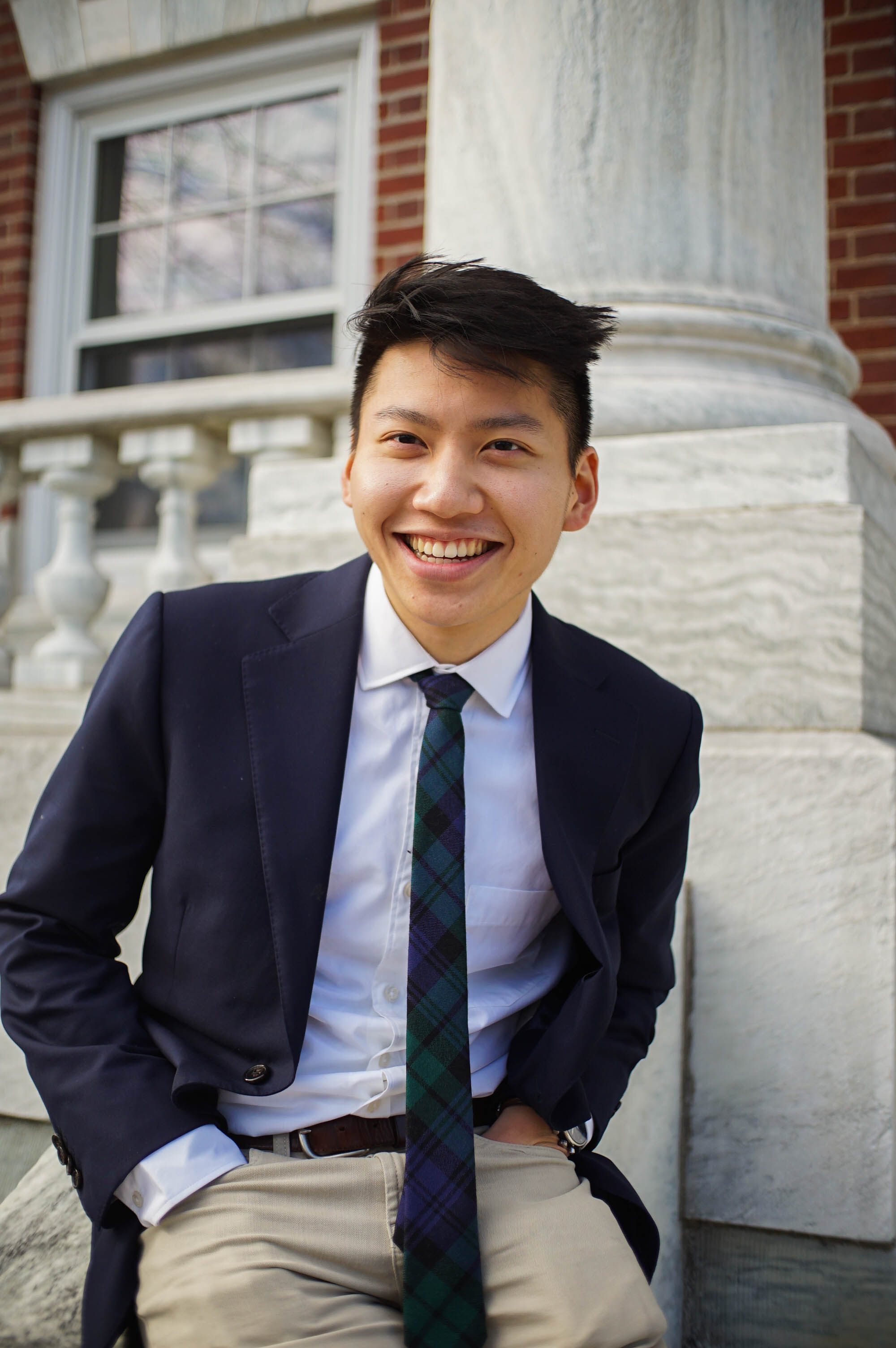
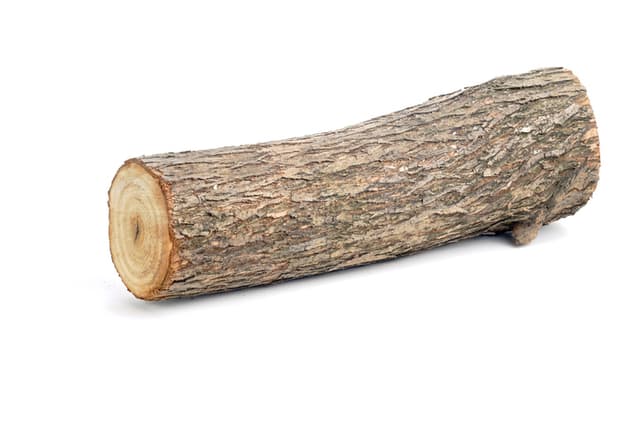
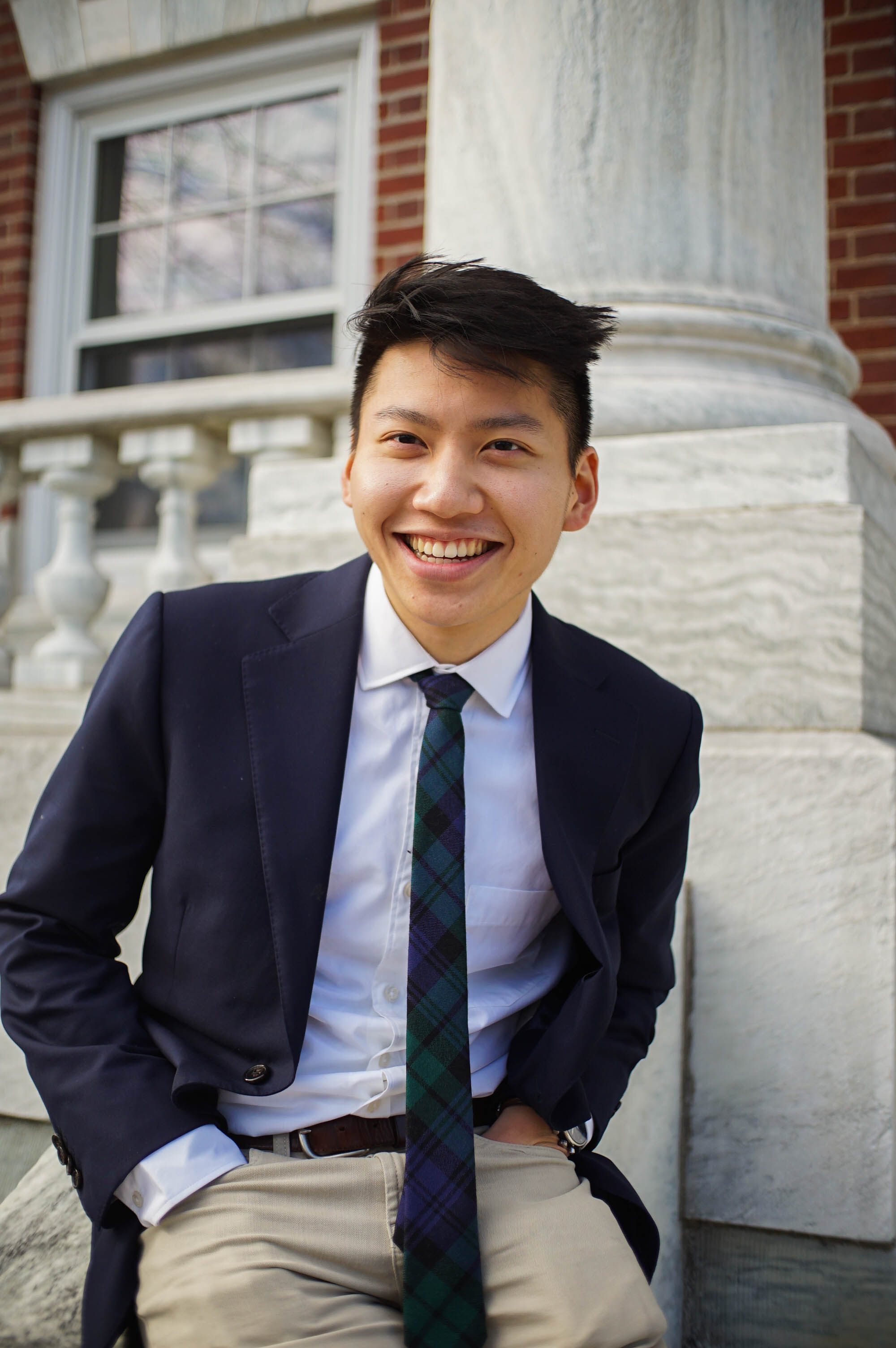
Linear Regression
The first thing to note is that if we are running a standard linear regression (using L2 loss), i.e. minimizing the sum of the squared residuals. This means that the residual vectors are orthogonal to the fitted line.
A perhaps easier way of visualizing this is to think of what is actually happening when we run a regression. The Y vector exists in Rn, and we have some column space spanned by all of our (independent) X vectors. What linear regression does is find a theta such that the residual after projecting the Y vector onto the the vector created by X*theta is minimized.
Projections and finding an orthonormal basis
In linear algebra we can project one vector onto another. The Gram Schmidt process is when we take a set of linearly independent vectors (which span some space) and create a set of c orthogonal normal vectors that span that same subspace. This is a process of projecting one vector onto the other repeatedly, to find orthogonal vectors, which is equivalent to taking the residuals after a regression.
It is also the case then, that we can think of regression as taking a Projection of Y onto the column space of X. The residual vector will be orthogonal to this projection, and therefore orthogonal to the column space of X, and therefore orthogonal to every column in X